Legacy tech companies will control enterprise AI
High customer acquisition costs and long sales cycles combine to make this a hard market for startups to penetrate
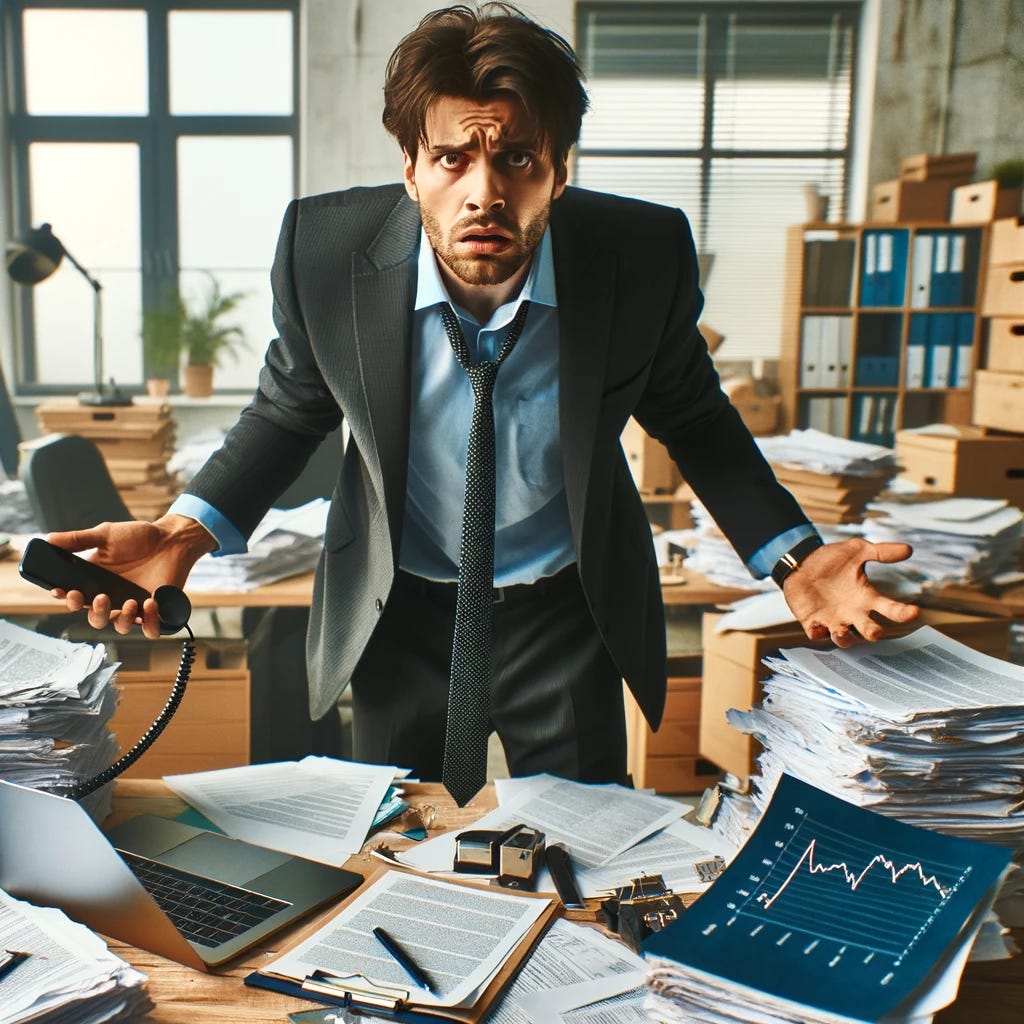
Introduction
One idea that makes venture capitalists salivate is enterprise AI. Imagine a large corporation, with tens of thousands of employees, installing an enterprise-wide AI tool. Those employees will be able to optimize their workflows, make their jobs more productive and efficient, etc. This is a gold mine, right? Finally, Silicon Valley’s plutocrats will be able to sell their Tahoe ski houses and buy a real chalet in Gstaad.
Not so fast. The world is a complicated place, and I think that a lot of venture capitalists, and the entrepreneurs in whom they invest, miss a number of things when they bet on enterprise AI. To the extent that enterprise AI is a thing (and I would agree that it is, or will be, a thing), it will mainly be controlled by the legacy megatech companies, for reasons we’ll explore later in this post.
Here’s tech analyst Azeem Azhar, talking about Groq1, which manufactures processors for high-performance AI computations:
Groq addresses one problem that both startups and large enterprises looking to scale their AI products: the cost of running models. As one anonymous founder said, they only make money “if people don’t use the product.” I’m hearing that enterprises are facing sticker shock when they move from a proof-of-concept, for a few users, to widespread deployment across their orgs.
To add to the complications, we’re now in a phase where LLMs are one part of increasingly complex systems. Building valuable applications involves linking AI models with databases and other applications and even sampling multiple LLMs. These systems yield more robust results, but they also multiply costs and create a challenging headache due to the need to constantly update components within a rapidly evolving ecosystem.
For any firm, this volatility in innovation makes planning hard. Should you buy now? Or wait a bit longer, when prices come down? Should you build for scale straight out of the gate or risk unsustainable economics later down the line?
Let’s make this more concrete. Consider two very different, large, legacy companies: Coca-Cola and Disney. A quick Google search suggests that there are around 82,500 Coca-Cola employees worldwide, and about 225,000 Disney employees. Whether these numbers are accurate isn’t really of concern here: the point is that these companies employ tens of thousands of people across many hundreds of different kinds of jobs. Both Coca-Cola and Disney employ white collar knowledge workers and blue collar laborers. Selling enterprise-wide AI tools to these, and other similarly-sized companies, is a large market opportunity.
And, we can infer all kinds of AI-related use cases for both Coca-Cola and Disney employees. Their marketing personnel can use an enterprise-grade version of ChatGPT (or similar tool from another company) to help with marketing copy; their legal personnel can use an enterprise-grade legal LLM to review contracts en masse; their logistics operations can use AI-enabled devices on the edge (i.e., mobile devices imbued with AI) to optimize warehouse or theme park operations, etc. The applications for AI in a large corporate setting are as boundless as venture capitalists’ optimism.
But here’s the thing. Enterprise sales is tough. Enterprise sales is even tougher when you’re selling something which requires corporations to give you access to their sensitive internal corporate data. It’s one thing for a random Disney marketing analyst to use her discretionary budget to buy a ChatGPT subscription, in order to write better marketing copy. It’s quite another to propose that OpenAI train a LLM on Disney’s internal corporate data.
Enterprise sales cycles are long, and these sales cycles significantly impact a tech startup’s cash position. Understanding these impacts is crucial for strategic planning and maintaining a startup’s financial health. Here are the key effects:
Delayed revenue recognition: With long sales cycles, revenue from sales takes longer to materialize. This delay in revenue recognition can strain the startup’s cash flow, especially if it’s relying on these sales to fund operations and growth activities.
Increased customer acquisition cost (CAC): The effort and resources expended over a longer sales cycle increase the cost of acquiring each customer. Higher CAC means more cash is tied up in the process of acquiring a customer before any revenue is realized, impacting the company’s cash position negatively until the sales are closed and payment is received.
Operational and overhead costs: Long sales cycles require sustained investment in sales and marketing activities, such as presentations, demos, and negotiations, over an extended period. These operational costs accumulate over time, requiring the company to spend cash without immediate returns, thereby reducing cash reserves.
Cash flow management challenges: Predicting cash flow becomes more challenging with longer sales cycles due to the uncertainty of when deals will close. This uncertainty can complicate financial planning and may necessitate keeping a larger cash reserve to buffer against periods of low cash inflow, limiting the cash available for other growh opportunities.
Risk of market changes: The longer the sales cycle, the higher the risk that market conditions, customer preferences, or technology landscapes will change before the deal is closed. Such changes can lead to lost sales or the need for additional investment to adapt the offering, further straining the startup’s cash position.
Funding and investment considerations: Startups with long sales cycles may find it more challenging to attract investment because investors often look for quicker returns on their investment. This could necessitate raising more capital than initially planned to cover operational costs during the extended sales cycle.
But all is not lost! There are some standard plays to reduce these cash flow risks. Basically, you’ve got to do something which few startups are able to do successfully. You have to diversify your revenue streams and optimize your sales process. All while building a new product and searching for product-market fit. Easy. Consider doing some of the following things:
Diversify revenue streams: Introduce new products or services with shorter sales cycles to balance cash flow.
Make the sale process more efficient: Leverage tools and techniques to streamline the sales process, reducing the length of the sales cycle.
Introduce pricing flexibility: Offer flexible pricing models, such as subscriptions or leasing to encourage quicker customer commitment.
Build a better financial model: Develop a robust financial model that accounts for the long sales cycles, and plan cash reserves accordingly.
Seek strategic partnerships: Form partnerships that can offer financial support or co-selling opportunities to shorten the sales cycle.
Of course, not all of these strategies are available to startups, which, as mentioned above, are also busy building a brand new product and searching for product-market fit.
But that’s not all. Remember, in order for AI tech to be useful enterprise-wide, the AI has to have access to all the company’s internal, confidential, proprietary data. You want to be able to train an LLM on this data, in order to allow employees to make better use of it. That requires a lot of trust on the part of the corporation. And that’s where legacy tech companies have an edge that your plucky startup does not. No matter how much entrepreneurs and their venture capitalists argue, often correctly, that startups just move faster, large corporate enterprises don’t actually care that much about the speed with which you operate. They care about stability. They care about security. They care about regulatory compliance. They care about reputation. They care about reliability. They care about customer service. These are all things which a startup entrepreneur would earnestly claim she cares about. But can a startup really deliver on these promises, in the way that a legacy tech company, with thousands of other entreprise clients, and a bullet-proof balance sheet, can?
How a startup can thrive in this environment
All too may enterprise AI companies are pursuing a horizontal strategy, in which they sell AI compute to any and all large enterprises. But when you try to be everyone’s friend you end up with no friends.
Vertical plays are harder, but possibly more fruitful. If you specialize in a particular industry, you become much more knowledgeable about your customers and their problems. And you can make a more convincing argument that they should trust their precious data with you, the industry expert in applying AI to [industry], instead of the generic legacy tech companies who serve everyone from insurance companies to entertainment companies to manufacturing companies.
Consider two very different industries: healthcare and law. John Snow Labs serves the healthcare industry and Harvey serves the legal industry. They know their industry inside and out, they get close to customers, they learn all about those customers and their pain points, and they solve problems exclusively for those customers. (I don’t have a financial stake in either of these companies.)
Yes, there are startups which pursue horizontal customer strategies, and do so successfully. The canonical example would be Salesforce, at least when it was still a startup. But the way that these successes usually happen is that they focus on one industry, acquire a number of customers in that industry, and then expand horizontally, to adjacent industries. For example, you might sell a solution to wealth management firms, and then start to pick up actuarial firms as a complementary customer base.
Conclusion
The enterprise AI market is likely to be controlled by the legacy incumbents. Bloomberg’s Parmy Olson notes:
Generative AI startups can build their technology in two different ways. They can develop their own version of OpenAI’s GPT-4 or Google’s Gemini for instance, a so-called foundation model that requires hundreds of millions of dollars in investment. Or they can build on top of an existing model, which only needs tens of millions in investment and which the vast majority of AI startups do today.
In both cases, the prime beneficiaries are cloud-computing giants Microsoft, Amazon and Alphabet Inc.’s Google, and AI chip maker Nvidia Corp. "Right now all these startups take money from venture capital investors and give it to cloud companies and Nvidia,” says Rodolfo Rosini, CEO of chip company Vaire Computing. That’s why Nvidia has seen its shares more than double in the last year, putting it near a $2 trillion valuation.
One way to avoid this is to focus on a particular industry vertical, and to become the recognized expert in applying AI to that particular industry. Develop deep relationships with customers, understand their problems well, and build products which solve those problems. Horizontal AI is a generic solution, and generic solutions tend to become commoditized and controlled by companies with large balance sheets. Vertical AI is a tailored solution, and tailored solutions tend to be less-commoditized, and higher margin.
hi Dave this was good stuff and generally you are spot on. We have followed your advice whether through good luck or good strategy. For both of our products, we have a niche customer: discretionary asset management research staff and sell-side research staff. I’ve been on both sides of the business for 29 years and know the main pain points. We have two products one is a conference call RAG app that works better than the Big 2’s offerings. it’s a pro-sumer, PLG product that is so easy to use we never talk to the customer. They just try our free plan and convert to paid. I’ve seen both the Big 2’s offerings in action and they’re poorly executed. The Big 2 will get there eventually, but in the meantime we have a window. In our enterprise app, we are working to wholesale it with a global consultant that will retail it for us to their global asset management clients. You also said that partnering with distribution is a good go to market strategy. There is a lot more to unpack here, but so far things are working. Our mission is to make all analyst’s and portfolio manager’s lives better one app at a time. We make apps this persona loves. All my best, Drew.