AI possibilities at the edge. Also: the broken VC industry
Interesting things start to happen when you imbue the edge of computer networks with intelligence. Also: the VC industry is broken
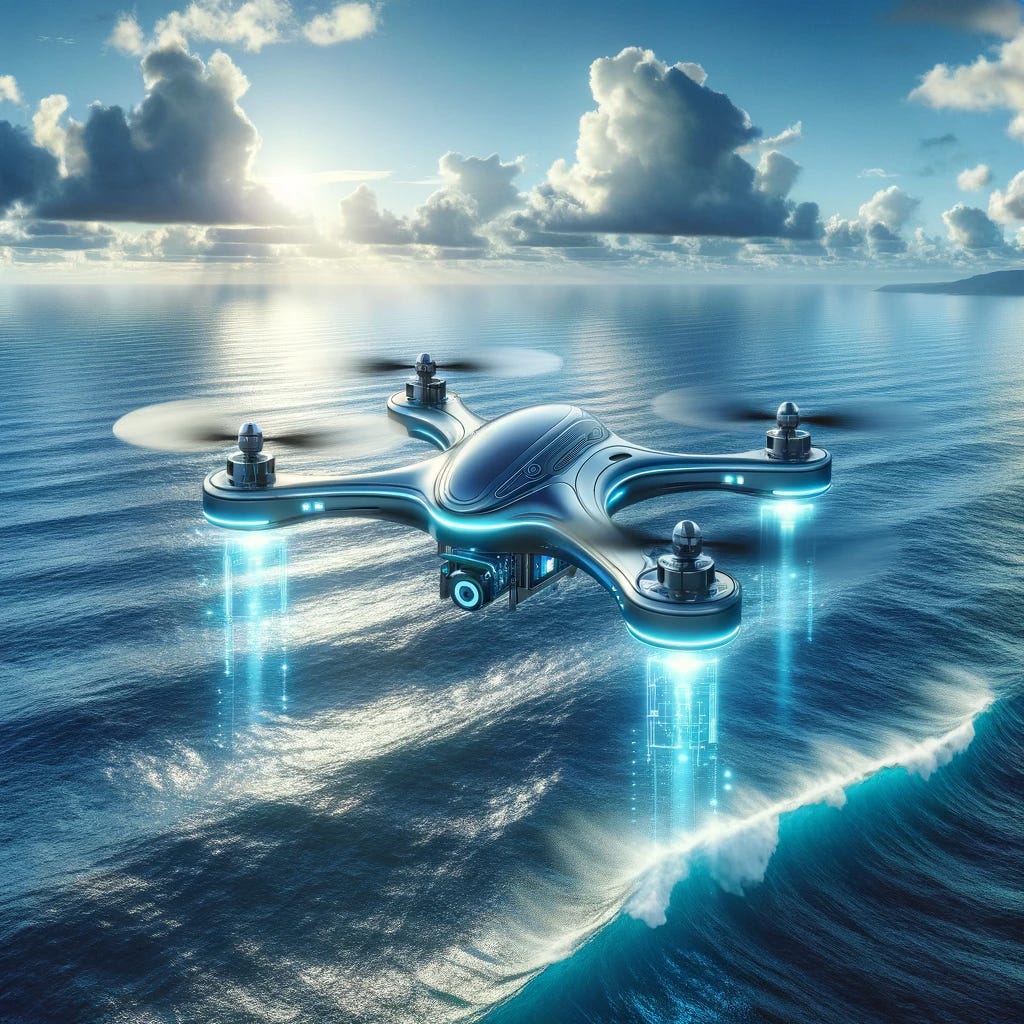
Intelligence at the edge
The conventional model for someone using AI goes something like this: the user fires up an app on her phone, or loads a web site on his computer, and interrogates ChatGPT or Claude or Midjourney. After some computation, in the background, in a data center far from the user, the AI tool dutifully returns a response to the inquiry. The response may be sublime or it may be hallucinatory, but it is a response delivered from afar by a server that the user has never set eyes upon. It is, in a word, a centralized computing experience. And, there’s a lot to be said for centralizing computational resources. ChatGPT 4, when it works, is the most powerful AI tech currently available to the public.1
But there’s a much different model for computational intelligence, and it’s this: the intelligence is instantiated in the device you hold. We’re talking, of course, about intelligence at the edge of computer networks. There are a number of reasons why this is so important, ranging from no latency to not requiring an internet connection. Imagine industrial sensors or drones imbued with intelligent agents. Imagine a drone flying over a remote industrial site, being able to autonomously take action based upon data it receives from industrial sensors. Imagine that all of this happens without having to rely on an internet connection to send the data back to a data center. Imagine not having to wait for a response from the remote computational resource.
This is what will happen when the edge of computer networks are imbued with intelligence. These use cases, and more, become available once we are able to store an AI on a mobile device. Here’s an article about some work on this goal:
An innovative approach to artificial intelligence (AI) enables reconstructing a broad feild of data, such as overall ocean temperature, from a small number of field-deployable senosrs using low-powered “edge” computing, with broad applications across industry, science and medicine.
“We developed a neural network that allows us to represent a large system in a very compact way,” said Javier Santos, a Los Alamos National Laboratory researcher who applies computational science to geophysical problems. “That compactness means it requires fewer computing resources compared to state-of-the-art convolutional neural network architectures, making it well-suited to field deployment on drones, sensor arrays and other edge-computing applications that put computation closer to its end use.”
The article discusses a paper, available here. The paper notes:
With the advent of widespread access to satellite data and cheap sensors, we have an opportunity to address several problems in Earth sciences and engineering in a manner not possible before. However, these powerful data sources are typically sparse, and leveraging them requires specialized approaches that can map the measured local data to the physics of the global field under observation. The limitations of current approaches introduce large uncertainties in myriad applications such as aviation safety, forecasting accuracy in adverse weather predictions, migration patterns of wildfire, contaminant tracing and tracking sequestered CO2 plumes. Having a general class of algorithms that is able to estimate and reconstruct the global field from sparse, local measurements will be a major advancement in this field. In this work, we present an efficient and effective deep learning approach to reconstruct fields from such sparse measurements.
Based on this conclusion, we can come up with many different applications for intelligent computing at the edge:
Smartphones: Enhanced personal assistants capable of context-aware suggestions, advanced image and speech recognition, real-time language translation, and personalized content curation.
Drones: Autonomous navigation for delivery drones, environmental monitoring, and precision agriculture. AI can enable real-time analysis of aerial imagery for tasks like crop health assessment or search and rescue operations.
Wearable Health Devices: Continuous health monitoring with predictive analytics for early detection of anomalies or diseases. AI can interpret data from sensors to provide health insights and emergency alerts.
Automotive: In self-driving cars, AI at the edge can facilitate real-time decision-making for navigation, obstacle avoidance, and driver assistance systems. Also, in regular vehicles, it can enhance safety features and optimize vehicle performance.
Industrial IoT: Predictive Maintenance in manufacturing equipment, where AI analyzes sensor data to predict and prevent equipment failures. AI can also optimize energy usage and streamline manufacturing processes.
Smart Home Devices: AI-drive home automation for energy management, security, and personalized experiences. This includes intelligent HVAC systems, security cameras with facial recognition, and smart appliances.
Retail: AI in edge devices can enhance the shopping experience through personalized recommendations, inventory management, and customer behavior analysis. Smart mirrors and AI-driven checkout systems are examples.
Agricultural Sensors: AI in agricultural sensors can optimize irrigation, detect pest infestations, and provide insights for crop yield enhancement.
Security Cameras: Real-time analysis for threat detection, facial recognition, and behavior analysis without the need to stream all data to a central server.
Smart Cities: Traffic management systems using AI to optimize traffic flow, reduce congestion, and improve public safety. Also, waste management systems that predict collection needs.
Environmental Monitoring: AI in sensors for monitoring air quality, water quality, and other environmental factors, providing real-time data for pollution control and environmental protection.
Robotics in Logistics and Warehousing: AI-driven robots for inventory management, sorting, and transporting goods. This can significantly improve efficiency and reduce human errors.
Edge-Based Content Curation: For media streaming devices, using AI to curate content based on user preferences and viewing habits, all processed locally for quicker response and privacy preservation.
Education and Training: Personalized learning experiences where AI adapts to the student’s learning style and pace, potentially even monitoring attention and engagement.
Energy Management: In small grids, AI can predict energy demand, optimize distribution, and integrate renewable energy sources effectively.
Of course, we could go on for quite a while coming up with other applications of AI-enabled edge computing. But you get the point: there’s a lot of really cool things we can do once the edge has become intelligent.
The broken VC industry
wrote a long, and very interesting, analysis of the current state of the VC industry. It’s hard to extract a good quote from the piece, but the gist is that the VC industry is beset by two competing factions: the Cottage Keepers and the Capital Agglomerators. A traditional venture capital firm like Benchmark is a Cottage Keeper, in this model, and an upstart like A16Z is a Capital Agglomerator. Back when VC was a much smaller industry than it is today, the Cottage Keepers ruled the roost, and generated steady returns for a small group of general partners (GPs) and their limited partners (LPs).
However, Capital Agglomerators, like the aforementioned A16Z, tried to do something different with venture capital. These funds wanted to offer complementary services for the entrepreneurs in whom they invested, in order to give these entrepreneurs a greater chance at success. While this sounds nice in theory, in practice it required much larger funds and much larger staff. VC funds were no longer lean operations. This meant that assets under management (AUM) increased commensurately. Larger AUM meant that returns, in the form of liquidity events (IPO or M&A) had to increase in proportion.
And this basically hasn’t happened. A combination of higher interest rates, a volatile stock market unreceptive to IPOs, and regulatory impediments to M&A have made liquidity events in VC land rare to non-existent over the past few years.
The VC industry faces a number of headwinds, and it needs to figure out a workable model for the future. This is especially the case if interest rates end up staying higher for the foreseeable future, as Howard Marks expects they will.
I’ll conclude with this observation from Chamath Palihapitiya.
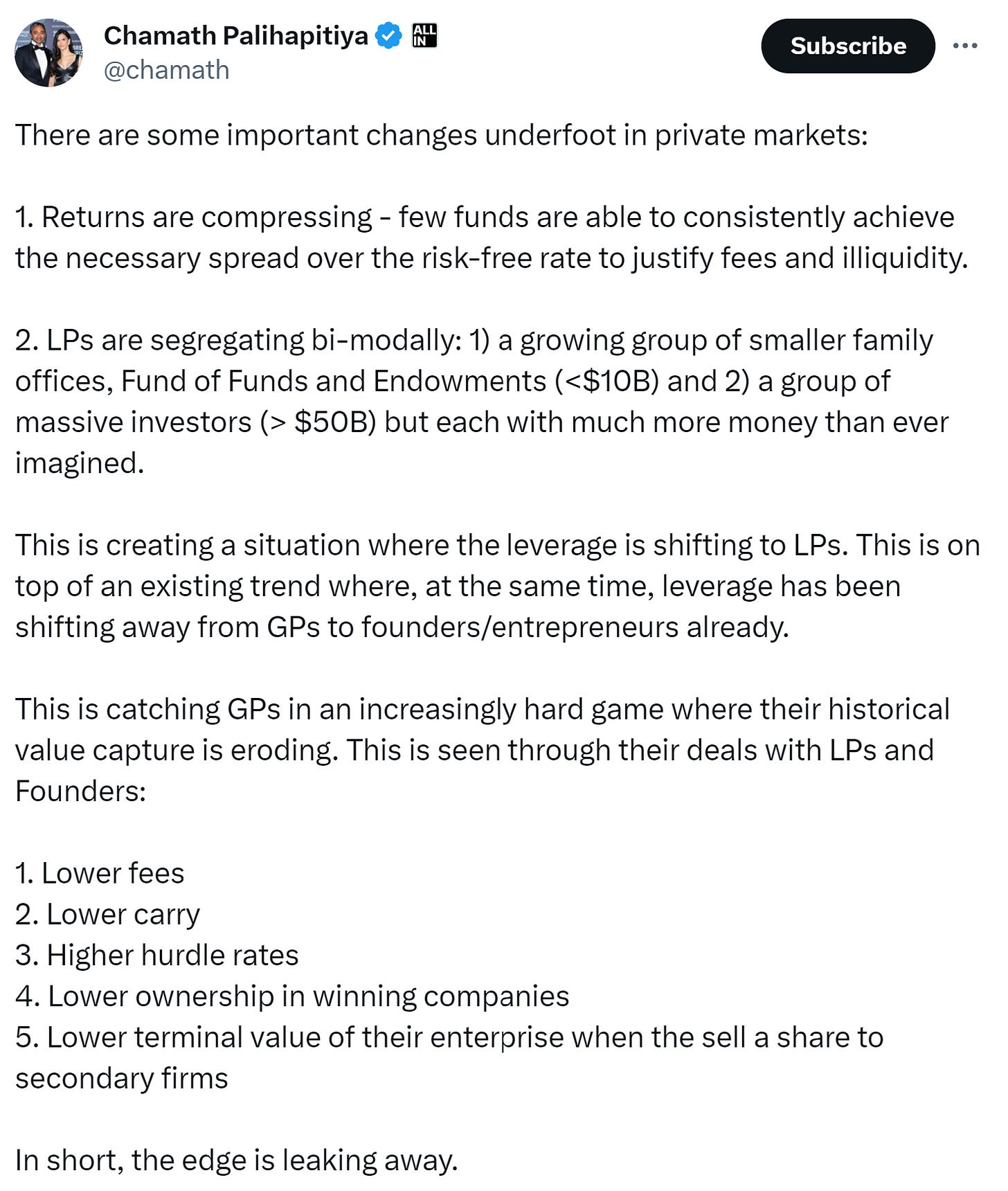
There may be more powerful tech available within OpenAI or Google or similar organizations, but if these more powerful tools do exist, they’re obviously not available to our hapless user.