OpenAI and the commoditization trap
As token costs for LLMs decline toward zero, fundamental models increasingly look like commoditized technology. How can OpenAI and its competitors avoid this trap?
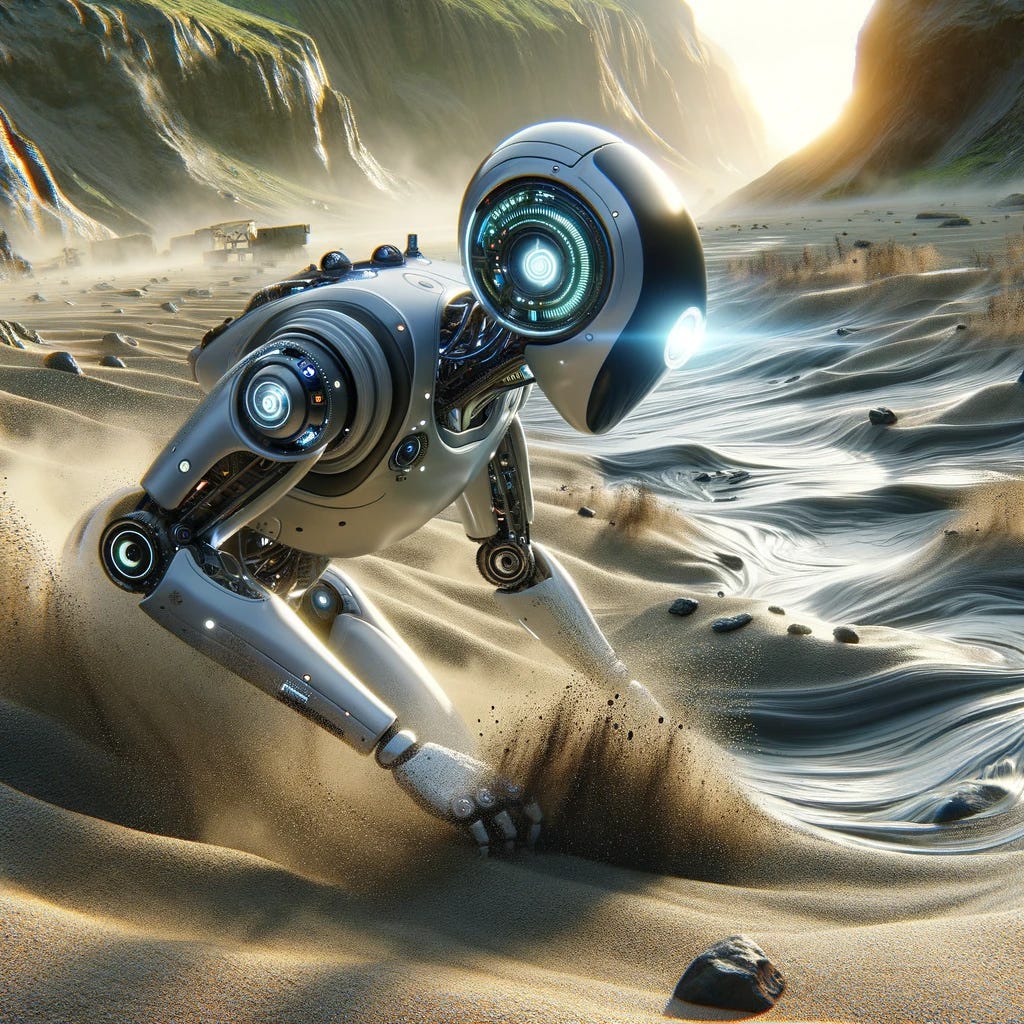
The greatest risk for OpenAI is not that it fails to develop artificial general intelligence or that it fires Sam Altman again. No, its greatest risk is that it can’t escape the technology commoditization trap. If OpenAI falls into this trap, it will compete with other AI companies solely on the basis of price, with nothing to differentiate its products from those of its competitors. When competitors compete solely on the basis of price, it’s a race to the bottom. Customers benefit, but companies find that they they’re hard-pressed to innovate or to develop new, more sustainable, revenue streams. OpenAI and its competitors will have to find a way to avoid this trap.
Commoditization refers to the process by which goods or services become indistinguishable from others in the same category, leading to competition based primarily on price rather than on differentiating features or quality. Elsewhere in the technology sector, certain types of computer memory and processors have become commoditized, leading to price being the main competitive factor. Indeed, one of the main reasons that Intel spent so much money on advertising its “Intel inside” slogan during its heyday was that it needed to compete with other suppliers on something other than product differentiation. Nvidia is generally seen to be the leader in the graphical processing unit (GPU) market, of which artificial intelligence companies are a major customer. Nvidia is, similar to Intel in an earlier era, doing everything it can to ensure that it doesn’t become commoditized.
In the field of artificial intelligence, we’re seeing commoditization occur with large language models (LLMs). LLMs are the things that companies like OpenAI build to allow other services, like ChatGPT, to operate on top of them. LLMs are frequently called foundational models. One useful way to think about LLMs is that they’re the substrate on top of which many interesting AI applications are built. Other companies that build similar technology include: Google, Meta, Anthropic, Mistral, and many more. While OpenAI’s LLM GPT-4 is the most powerful and capable one, the cost that users pay to use any of these LLMs is nearly at parity. One source showing the costs across major LLMs is here. Costs vary by fractions of a penny.
The smallest amount of data that these LLMs process is called a token. And users pay on a per-token basis. When token costs across all major LLMs are at or near parity, the market isn’t differentiating between any of the companies: this is commoditized tech. When tech is commoditized, companies have no pricing power, and their revenues are at risk. The solution is to develop ancillary revenue streams whose pricing is sustainable. And, given its reported $85 billion valuation, OpenAI needs to develop some significant revenue streams. We have all seen what has happened to ther highly valued tech startups whose revenues can’t justify their high valuations.
As technology matures and becomes more widespread, differentiation becomes key to maintaining a competitive edge. When similar products or services are offered at comparable prices, pricing power is eroded, and potentially, the profitability of the companies involved. This is a critical concern for any company, particularly for those like OpenAI, which operate in a rapidly changing industry.
Given all of this background, what can OpenAI and its competitors do, to ensure that they are able to grow their revenues, and not fall into the commoditization trap? To address this issue, OpenAI and its competitors can consider several strategies:
Innovation and differentiation: Continuously improve the technology to offer superior capabilities compared to competitors. This could involve more advanced language models, faster processing times, unique features, or better integration with other technologies. OpenAI’s ongoing research and development could focus on creating unique, high-value features that are difficult for competitors to replicate. The risk with this strategy is that the competition is relentless and intense. You’re one bad product cycle from being eclipsed by your competitors.
Custom solutions for enterprise clients: Tailoring products and services to the specific needs of large enterprise clients. This could involve custom-built AI solutions for different industries, such as finance, healthcare, or manufacturing. Bespoke solutions can command higher prices due to their tailored nature and the value they bring to the client’s specific context. Risks here include long sales cycles, needing to build a sales operation, and competing with experienced enterprise services providers such as Snowflake, Databricks, and other companies looking to sell AI-related services to their existing customers.
Diversification into new markets or products: expanding the range of products and services beyond large language models. This oculd include ventures into different aspects of AI, like computer vision, robotics, or AI ethics and safety tools. Diverisifcation can reduce the reliance on a single product line and open up new revenue streams. On the other hand, diversification can also detract attention from one’s core business model, and force company executives to spin their wheels on unproductive efforts.
Partnerships and collaborations: Forming strategic alliances with other companies to create new products or enter new markets. This could involve partnerships with companies in different sectors, leveraging OpenAI’s AI capabilities to enhance their products or services. Risks here include spending time and money pursuing partnerships that are a poor fit, or selecting unreliable partners.
Platform approach: Developing a platform that enables third-party developers to create and sell their applications. This approach can create an ecosystem around OpenAI’s technology, driving usage and a percentage of revenue from third-party offerings. OpenAI is trying to build this ecosystem with its GPT store, which is similar to what Apple’s App Store does for its iOS ecosystem. It remains to be seen whether this iniative succeeeds.
Monetizing data and insights: Given the vast amount of data processed by LLMs, there could be opportunities to monetize insights derived from this data. This is a tricky thing to pull off well: there a lot of privacy-related issues to consider, and OpenAI (or similar company) could risk severe public backlash for improper use of personal information.
Subscriptions and premium services: Offering subscription-based access to advanced features or premium services. This could include higher processing speeds, more extensive data sets, or advanced customization options. OpenAI obviously offers this with its ChatGPT Plus and Team plans. It’s not publicly known how many ChatGPT Plus and Team subscribers OpenAI has, or what percentage of their overall revenues these subscriptions comprise.
Educational and training programs: Providing educational services and certification programs around AI and machine learning could be a revenue stream. This can help in creating a knowledgeable user base, potentially leading to better and more innovative uses of the technology. The problem with this strategy is that corporate training and education’s isn’t an especially lucrative or scaleable opportunity, relative to others available to software firms.
While the risk of commoditization is real, there are multiple avenues for OpenAI and its competitiors to explore for developing sustainable and differentiated revenue streams. The key is continuous innovation, adaptability, and a deep understanding of the evolving market and customer needs.
Given the potential commoditization of large language models, and the challenge of sustaining high valuations in the tech industry, it is crucial for companies like OpenAI to develop effective strategies to maintain their competitive edge and financial viability. The strategies mentioned above are a good guide for any company looking to escape the commoditization trap. But what are some concrete things that a company can do with these strategies? What kinds of revenue streams will allow a company to avoid falling victim to commoditization? It’s important to remember that, while the underlying technology, in this case, large language models, like will become commoditized, there are opportunities that these companies can pursue which will provide some insurance against the perils of commoditization:
Specialized AI consultancy services: OpenAI could offer consultancy serices that leverage its expertise in AI. These services could include AI strategy development, implementation support, and optimization for businesses. By offering high-level consultancy, OpenAI can capitalize on its unique insights into AI applications across various industries.
AI-as-a-Service (AIaaS): Develop an AI-as-a-Service platform where businesses can access OpenAI’s cutting-edge tools and technologies on a subscription basis. This model allows for scalable and flexible use of AI resources, tailored to the needs of different businesses and industries. OpenAI’s ChatGPT service is a useful model here, but it likely could be enhanced for enterprise clients.
Exclusive research partnerships: Forming exclusive partnerships with leading research institutions and corporations for the development of next-generation AI technologies. These partnerships could focus on breakthrough innovations and have the dual benefit of generating revenue and keeping OpenAI at the forefront of AI research.
High-value licensing agreements: Licensing proprietary AI technologies and models to other businesses can be a significant revenue stream. These agreements could be particularly valuable in sectors where advanced AI capabilities provide a competitive advantage, like autonomous vehicles, healthcare diagnostics, or financial forecasting. OpenAI already has a partnership with Microsoft, and it’s unclear whether that partnership constraints their opportunities for similar partnerships with other companies.
Inventing in AI startups: Creating a venture arm to invest in promising AI startups. This approach not only potentially generates significant returns but also keeps OpenAI connected to the latest innovations and emerging trends in the AI space. This is corporate venture capital, a tried-and-true method for large companies to keep abreast of entrepreneurial developments adjacent to their business interests. While these arrangements often look good on paper, in practice, it’s not clear how much value accrues to the parent corporation via corporate-sponsored venture capital.
Developing proprietary hardware: Exploring the development of specialized hardware optimized for running OpenAI’s models. This oculd include AI processors or custom server architecture, which could be sold or leased to businesses running intensive AI operations. There have been recent news reports about Sam Altman soliciting investors for custom chips for OpenAI, for example.
AI-enhanced products and services: Developing new products and services that integrate AI in innovative ways. This could range from AI-driven content creation tools to advanced analytics platforms. The risk here is that it takes a while to find product-market fit for any new product.
Global AI competitions and challenges: Hosting high-profile AI competitions and challenges with substantial prize pools. These events can drive innovation, attract talent, and generate significant media attention and sponsorship revenue.
Strategic acquisitions: Acquiring companies that have complementary technologies or that can help OpenAI enter new markets. This could include companies specializing in different AI domains, data analytics firms, or even companies with large, valuable datasets.
The bottom line is that the underlying technology which powers OpenAI and its competitors, large language models, is likely to become commoditized in the near future. Token costs are rapidly declining to zero, and the market isn’t indicating any differentiation amongst the various service providers. OpenAI and its competitors need to develop new revenue streams with sustainable pricing power, in order to avoid falling victim to commodization. Many other technology companies have successfully navigated this risk, despite selling commoditized products and services. There’s nothing stopping OpenAI or its competitors from also successfully avoding this trap.