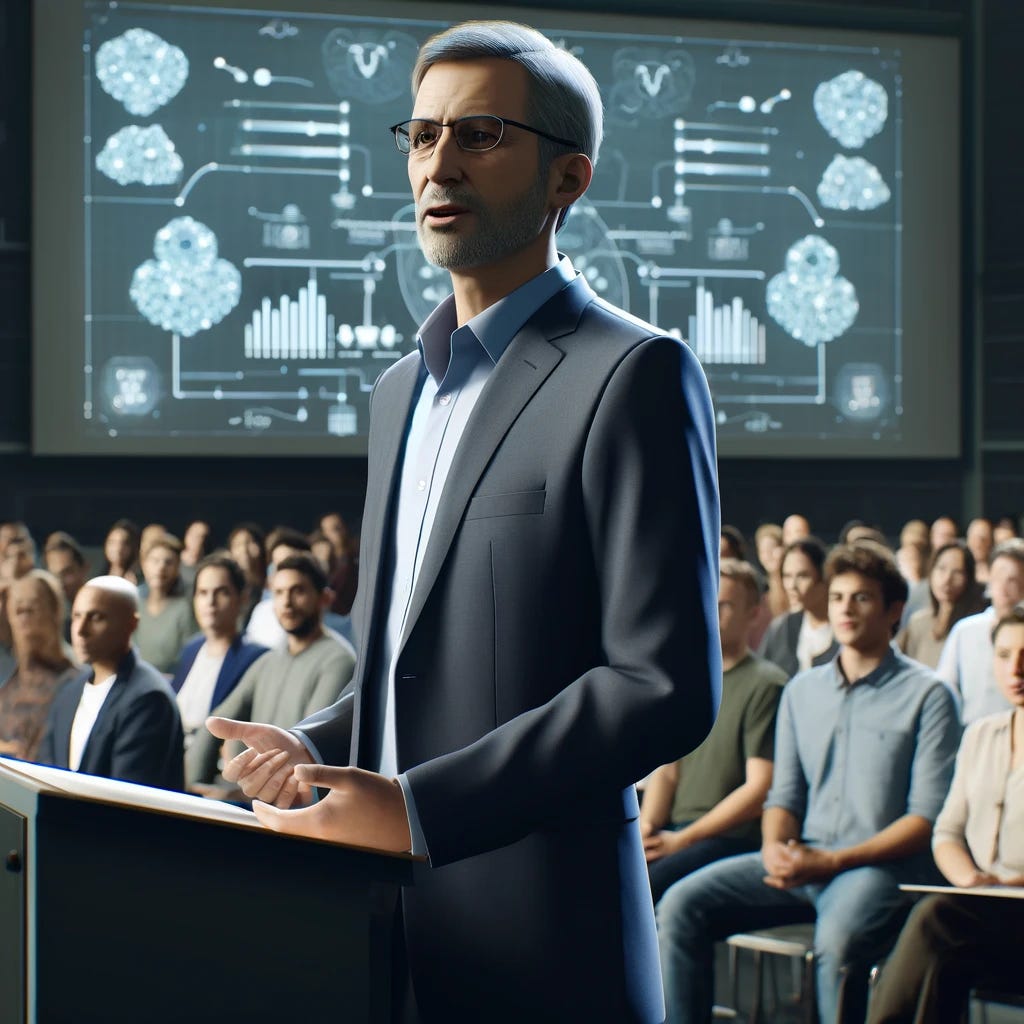
Expertise is a double-edged sword. It might generate demand for your time, but it can also blind you to developments which refute your priors. This is especially true in fields as volatile as technology. Consider the case of the IT executive who I saw speak when I worked at Citigroup before the Global Financial Crisis. The executive was asked by another employee what Citi’s cloud computing plans were, and the executive bitterly derided Silicon Valley and its then-nascent obsession with cloud computing. No “serious business like a bank,” we were sternly told, would ever trust its computing needs to a distant cloud computing service. From the perspective of 2024, this claim is, of course, risible. But here was an expert, confidently holding forth about his expertise in enterprise computing. From his perspective, cloud computing was as ephemeral as clouds themselves.
It’s worth considering this story in the context of all the experts who are skeptical about the rise of AI. What priors are they relying on, and how might we refute those priors with observations in the field? Experts who have deep knowledge about a field tend to underestimate the potential of new developments. They are very aware of the complexities and challenges in their field, which can lead to skepticism about major breakthroughs.
To counter this, it’s important to look at objective measures of AI progress rather than just experts’ opinions. The rapid improvements in AI performance on benchmarks like ImageNet, the ability of large language models like GPT-4 to engage in open-ended dialogue, and the expanding real-world applications of AI are strong evidence of transformative progress.
Experts’ views are shaped by their past experience and the state of technology during their formative years. The Citigroup IT executive, whose story I related earlier in this piece, dismissed clooud computing because it violated his prior belief that “serious businesses” like banks would never outsource computing. His view made sense based on the technology of the past, but it failed to account for how rapidly cloud computing technology was advancing.
Similarly, AI experts today may be anchored to the limitations of earlier AI systems, and they may be skeptical of large language models (LLMs) because LLMs’ broad set of use cases violate the paradigm of narrow, specialized AI systems that have dominated in the past. Looking at the actual results being produced by today’s AI systems, rather than relying on outdated assumptions, is key to overcoming skepticism.
Experts also have incentives and biases that predispose them to being AI skeptics. Academics who have built their careers around classical AI approaches have a vested interest in their paradigm. Big tech executives want to downplay the disruptive potential of AI due to concerns about regulation. Even well-intentioned experts may subconsciously resist the notion that AI could automate many knowledge work tasks.
The solution is to look for evidence of AI progress that comes from objective measures and real-world applications, not from experts’ statements. Put more weight on demonstrations than opinions. And consider the track record and incentives of experts when evaluating their claims.
Finally, it’s crucial to recognize that progress in AI is happening exponentially, not linearly. Exponential trends are notoriously hard for humans to grasp. What seems like a distant, speculative possibility can become reality much faster than expected. Many AI experts have spent their careers in an era of slow, linear progress in AI capabilities. So they may have a hard time internalizing that we’ve now entered an exponential phase. Studying the history of exponential technology trends, and being open to the possibility that the future may be very different from the past, is essential for recognizing the transformative potential of AI.