AI & exponential growth: what does it all mean?
AI is growing at an exponential rate but a lot of people don't really understand what that means.
We frequently see news articles and blog posts which claim that AI technology is becoming exponentially more powerful over time. The implications of exponentially improving technology, especially if sustained over a long period of time, are rather profound. It seems pretty important, therefore, to develop an intuition for what “exponential growth” means. Because we so infrequently see exponential growth in our daily lives, most people don’t have a great intuition for it. The goal of this post is to provide a framework for developing an intuition about exponential growth.
First, let’s take a look at a few articles which discuss the exponential growth in AI technology capabilities. This will provide us with a base line for understanding the claims being made.
Charted: The Exponential Growth in AI Computation
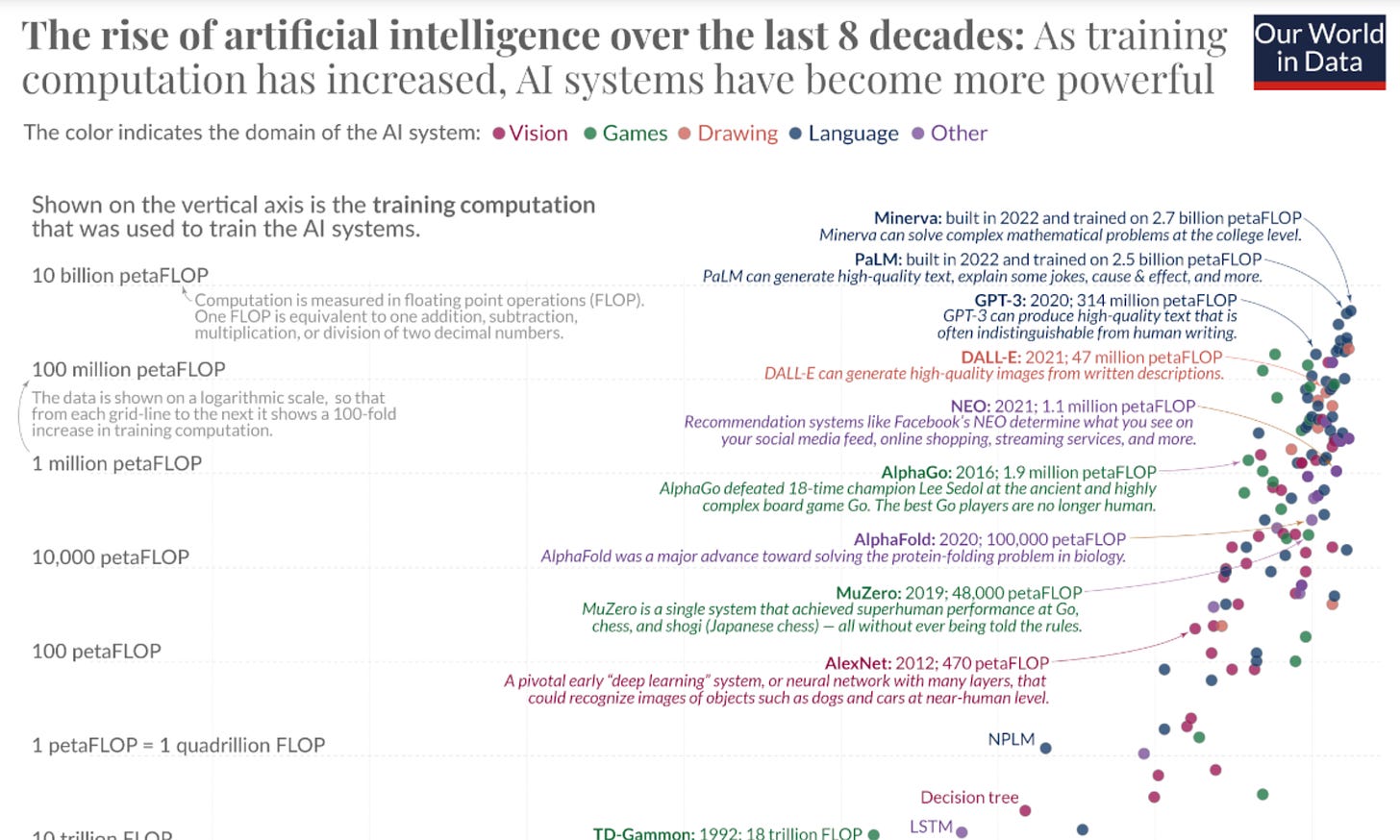
This image, especially the full, high-resolution version, provides a great graphical representation of the exponential growth in AI capabilities over the past few decades. A naive extrapolation of the graph into the future portends a much different world than the one we presently live in.
AI research began in the 1940s, however, we’ve only recently seen significant exponential growth in capabilities. We track this growth via the computational power used to train AI models. We can divide the growth trajectory into three eras: Pre-Deep Learning (1950-2010), Deep Learning (2010-2016), and Large-scale models (2016-2022). Each era reduced the time required to double computational power, from 18-24 months in the Pre-Deep Learning era to just 11 months in the Large-scale models era.
Growth in Artificial Intelligence is Beyond Exponential
Traditionally, AI computational power doubled approximately every two years, in line with Moore’s Law. However, since 2012, this growth has dramatically accelerated, doubling every 3.4 months, far exceeeding Moore’s Law. This growth trajectory is often depicted as a “hockey stick” graph due to its steep inline. Alternatively, it’s referred to as a “j-curve”.
AI’s rapid improvement has led to significant advancements in various fields. For instance, AI is now instrumental in drug discovery, analyzing billions of compounds to find potential new treatments. It’s also enabling autonomous driving technology and augmenting military capabilities. One example is an AI winning a dogfight against a human F-16 fighter pilot, a feat achieved through 4 billion simulations.
The Science of Machine Learning
The factors which allow for exponential growth in AI capabilities include: increased computational power, availability of vast data sets, advances in machine learning algorithms, increased investment and research, democratization of AI tools and frameworks, and interdisciplinary integration. This has created a positive feedback loop, propelling AI growth at an unprecedented rate.
Developing an intution for exponential growth
Given all of this, why do so many people have trouble understanding exponential growth? How can we develop a better intuition for it, in order to understand what is happening with AI technology today? Understanding this stuff is important because the growth rates we see with AI technology suggest that our near term future will be much different than the present world in which we live. And that’s a hard concept for many to wrap their heads around.
Linear Thinking Predominance
Humans are naturally inclined towards linear thinking, where we expect changes to happen in uniform, steady increments. This is largely because many everyday experiences and observations (e.g., the way we perceive aging, speed, or distance) tend to be linear. Exponential growth, with its rapid and escalating progression, doesn’t align with this linear perspective.
Difficulty in Grasping Large Numbers
Exponential growth often involves very large numbers. Human brains are not well-equipped to intuitively grasp or visualize large numbers and the scale of their increase, especially when this growth happens over a short period. There is the parable of rice and a chessboard, in which a king offers a wise man a reward. The wise man requests one grain of rice be placed on one square of the chessboard, and that the amount of rice be doubled each day, until all 64 squares are covered with rice. Naively, this sounds like a reasonable compensation scheme.
However, when you look at the math, you get a much different picture. The formula for the number of grains on the 64th square is 2^(64-1) = 2^63
. Total grains, though, is the sum of the grains on the 64th square, and the grains on the 63 preceding squares. Mathematically, this is a geometric series:
where a
is the first term (1 grain) and r
is the common ratio (2, or a doubling from one square to the next). If we sum this series of numbers, it resolves to 2^64 - 1
. This results in an immense number, far exceeding the world’s production of rice. Exponential growth, even starting from a small base, can lead to unimaginably large numbers.
Underestimating Compounding Effects
Exponential growth is fundamentally about compounding, where each step is significantly larger than the previous ones. People often underestimate the power of compounding, especially over longer periods. The dramatic escalation in later stages of exponential growth comes as a suprise because the initial stages might appear deceptively slow or insignificant.
Consider the parable of the rice and chess board, discussed above. Intuitively, you start with a small number of grains (1). Consider the first eleven numbers in this sequence:
1
2
4
8
16
32
64
128
256
512
1024
That’s the first eleven squares, and summed together, it’s less than 2,000 grains of rice. 2,000 grains of rice isn’t much, and we’ve already covered 17% of the chess board! Surely this is a manageable amount of rice. And yet, once you do the math, you see how very wrong this intuition is.
Cognitive Biases
One of the most common objections to AI that I see is that it is overhyped. A user will prompt ChatGPT, ChatGPT returns a hallucination or something that is otherwise unreliable, and the person concludes that AI is yet more Silicon Valley hype. Therefore, this person concludes, there is nothing worth paying attention to when it comes to AI, and any claims about exponential growth can safely be dismissed.
There are two cognitive biases at work here.
First, there’s the anchoring effect, in which one relies too heavily on the first piece of information seen. If you prompt ChatGPT, and it returns a hallucination, the anchoring effect will induce you to assume that all future interactions with ChatGPT will be equally fruitless. People do this because they assume that ChatGPT and similar AI tools are deterministic. Of course, they’re not: they’re non-deteriministic, which means sometimes they act in surprising ways. And one of those surprising ways is hallucinations. This takes time to get used to, and there is a learning curve to learning how to prompt well.
Second, there is the availability heuristic, in which one bases judgment on readily available information. If all you know about AI is that “ChatGPT confidently hallucinates bullshit” then what reason do you have to pay attention to it? A confident bullshit generator can be found in every bar in America.
These biases lead people ignore underlying growth rates, in favor of lazy reasoning.
Lack of Direct Experience
In order to develop an intutive understanding of the rate at which AI technology is improving, you have to have extensive experience using the technology. And, it is easier to just go about your life, and assume that the biases you hear from other people, for which, see above, are an accurate view of the state of the world.
It is only with direct, hands-on experience, that most people develop an intuition for the extraordinary gains we see in AI capabilities.
Complexity in Conceptualization
Exponential growht is abstract, and a lot of people simply have trouble reasoning about abstract concepts. To some extent I think you can train yourself to be able to reason well about abstract things. But since daily life doesn’t really require that you develop this skillset, it’s something that you have to want to do. And many people simply don’t want to do it, or are not even aware of the distinction between abstract and concrete concepts.
Misleading Early Stages
Recall the example of the rice on a chessboard. In its first eleven squares, we only get to 1,024 grains of rice. This is a tractable number. And it doesn’t suggest anything like the final result of 2^64-1
grains of rice on the final square.
In its early stages, exponential growth can look very similar to linear growth, leading to misconceptions about its nature. The real difference becomes apparent only after several growth cycles, by which time the scale of change can be unexpectedly large.
Psychological Distance and Denial
When faced with the implications of exponential growth, especially in contexts like population growth, pandemics, or climate change, there’s a tendency for psychological distancing or denial due to the overwhelming or alarming nature of the outcomes.
Developing a better intuition for exponential growth often involves actively retraining our thought processes, seeking out and working through real-world examples, and learning to question our initial, linear assumptions. This is a challening but valuable skill, especially in a world increasingly shaped by technologies and phenomena that exhibit exponential characteristics.
Exponential growth is important, but also in the real world does have limits.
The rice doubling was ok, but then I was hoping for some example more ai related.
Others, like Brian Chau, note the limits of ai training growth, data available and computation costs.
Ai fighting bots & drones are likely at the 1 2 4 stages of improvement. Software can’t eat the physical world.